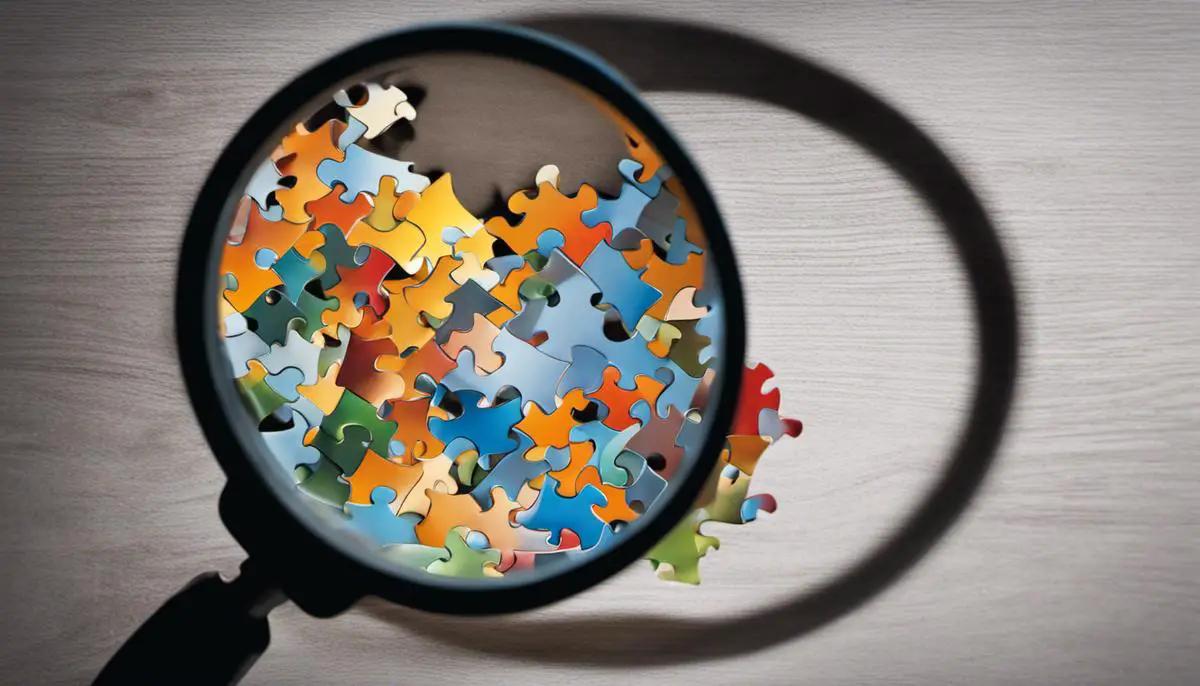
What are the confounding variables? This article comprehensively discusses this often encountered term in research and statistics.
Table of Contents
Introduction
In the realm of research and data analysis, the concept of confounding variables holds considerable importance. Their impact is significant as they have the potential to skew results, leading to potential misinterpretations of data. Understanding confounding variables, their role in research scenarios, and the techniques to control them are vital aspects for researchers in any field.
The purpose of this article is to explore the concept from a broad perspective, encompassing its understanding, the techniques to effectively control it, its practical examples, the repercussions of disregarding it, and real-life case studies that showcase its influence in research contexts.
Confounding Variables in Detail
The Role of Confounding Variables in Research: A Definitive Examination
Confounding variables can be defined as variables that influence both the dependent variable and independent variable, causing a spurious or erroneous association. This characteristic offers a perplexing complexity to research, for they meddle with the causal relationship that the experiment intends to establish, adding an extra layer of uncontrolled influences which might distort the conclusions drawn.
Confounding variables can be defined as variables that influence both the dependent variable and independent variable, causing a spurious or erroneous association.
Confounding variables are prevalent in all forms of research, whether that be in clinical studies, epidemiology, psychology, or social sciences, and manifest in many guises such as concurrent exposures, alternative causes, or even prognostic factors.
To understand the magnitude of confounding variables’ implication, consider a classic example.
In a study investigating the relationship between lung cancer and smoking, failing to account for age might lead researchers astray, drawing an incorrect conclusion that smoking is not related to lung cancer, while in reality, older persons often have a higher likelihood of having smoked for many years and having developed lung cancer.
As such, confounding variables, by their so-called ‘contaminating’ nature, can critically undermine the quality of the research. They can occlude the true relationships that exist between variables, leading to biased results and incapacitating the validity and reliability of findings. It is, therefore, of utmost importance that researchers employ robust strategies to control these elusive confounders.
Different methodologies exist to counteract their influence; one of the most prevalent includes matching, where researchers match the participants in different groups based on the confounding variables. Another approach is adjusting in statistical analysis, where researchers statistically control the confounding variable’s effect.
Moreover, in experimental research designs, researchers use random assignment to control confounding variables, such that each participant has an equal chance of being in any of the groups. This method diminishes the likelihood of shared characteristics amongst groups significantly and, therefore, reduces potential confounder interference.
It is essential to acknowledge that while these steps may control confounding variables, they cannot eliminate their existence entirely. As in the very crux of empirical research lies the human factor, a concoction of unavoidable and unalterable variables, a quest for absolute devoidance remains somewhat elusive. Instead, a continuous strive for sophistication in research methodologies remains pivotal in mitigating the confounding variables’ influence.
It suffices to conclude that the confounding variables bear undeniably profound connotations within the domain of research, not merely as factors to control, but also as flags for prospective growth and advancement in methodologies. Their presence urges researchers to develop increasingly precise and controlled systems, thus pushing the boundaries of invaluable knowledge production and exploration.
Statistical Techniques for Controlling Confounding Variables
Statistical Methods for Managing Confounding Variables
One potent method for handling the confounding variables is stratification—a tactic wherein data is split into different ‘strata’ or segments. By grouping data into homogeneous segments, we curtail the ability of inherent confounding variables to mar our results. Often employed in epidemiological research, stratification ‘levels the playing field,’ eliminating variance that can obscure the clarity of our findings.
Regression analysis is another vitally important method. This computational process allows us to put a leash on confounding variables, taking them into account when modeling the relationship of interest.
Factorial ANOVA (Analysis of Variance), peeks into the subsets of scientific research. It contrasts the means of two or more groups, considering the effect of multiple factors simultaneously. By examining group differences across several independent variables, it can sift out any hidden confounding factors.
Further, the propensity score (PS) method, a relatively recent addition, is gaining recognition. PS methodology employs a score for each test subject that reflects the probability of assignment to a particular group, dependent on their background variables. This approach allows researchers to match subjects across groups based on these scores, helping to control for confounding variables.
Mantel-Haenszel technique, hinging on stratification, is another revered tool in our statistical arsenal. Herein, the effect of a trivial variable is assessed through each stratum, and a summary statistic is accumulated. This method, especially beneficial in retrospective studies, sidesteps any convoluted confounding influences.
Finally, machine learning techniques are making waves in data analysis. Algorithms such as random forest and boosted regression offer the potential to unmask and minimize the confounding variables in ways traditional methods can’t.
Empowered with these statistical methodologies, we can shed light on the impact of confounding variables, one study at a time.
Examples of Confounding Variables
Early illustrations of confounding variables in research scenarios are drawn from multifarious instances, much like a profound network of science that is subtle yet unavoidable. One such classic confounding variable example comes from observational epidemiologic studies.
Let us reflect upon risk factor studies for lung cancer conducted in the 20th century.
Example 1: Does coffee increase the risk of lung cancer?
There was confusion about whether coffee may increase the risk of lung cancer or not. Several research scenarios revealed consuming coffee was linked to a higher risk of contracting lung cancer.
The confounding variable that surfaced, however, was smoking. Individuals who consumed coffee were more likely to smoke, a habit proven to indeed increase the likelihood of lung cancer.
Thus, smoking behavior was the confounding variable in this study, highlighting the misleading association between coffee consumption and lung cancer.
Example 2: Does advertising expand product sales?
A further example is centered on advertising research.
Should one attest digital advertising expands product sales? An upward trajectory in sales tracked in harmony with the implementation of digital advertising may lead to an ostensible association.
However, additional variables, including SEO tweaks, changes in the market, product quality improvements, or the addition of new distribution channels, can confound this apparent connection. These variables intertwine with the independent variable (digital advertising) and the dependent variable (product sales), obfuscating the true relationship at the heart of the study.
Example 3: Does classroom technological tools enhance students’ academic performance?
Now turn attention to a field starkly divergent: educational psychology. Picture research aimed to discover if classroom technological tools enhance students’ academic performance.
An initial study may ascertain that students in classrooms with advanced technological tools perform better in academic assessments. Yet, several variables could confound this finding. Such classrooms may be in wealthier areas where students have access to additional educational resources, including private tutors or intensive after-school programs. On such occasions, socioeconomic status, being the confounding factor, misdirects the actual correlative relationship between the two variables.
Each of these examples underlines the intricate labyrinth that is intrinsic to research. While the phenomena of confounding variables offer challenges, it is indeed a pioneering aspect that drives science as an avenue of continuous exploration and improvement.
The Impact of Neglecting Confounding Variables
Understanding the implications of neglecting confounding variables is paramount. These potentially impactful variables, as already scrutinized, can serve as formidable obstacles or catalysts for methodological evolution. Neglecting them can lead to severe and often unethical distortion of research findings — a pitfall that serious and conscientious researchers should strive to avoid.
1. Erroneous Conclusions
A direct impact is the distortion of research findings, potentially leading to erroneous conclusions. Erroneous conclusions, based on research in which confounding variables were not properly accounted for, may then be applied as the foundation for further research, creating a domino effect of error-prone studies.
Furthermore, these flawed findings may be employed in settings beyond research, such as healthcare decisions, designs and evaluations of interventions, or policy formulations. The potential real-world implications of such flawed decisions can be devastating or beneficially consequential.
2. Threat to Intellectual and Scientific Integrity
On a deeper level, neglecting confounding variables can erode the heart and soul of academia: intellectual and scientific integrity. The commitment to adhere to the rigorous, ethical practice of identifying, assessing, and controlling for variable confounders, is part of what defines an academic or scientific endeavor as reputable and worthy of esteem. To bypass this critical step could undermine the integrity of the research, leading to a loss of trust.
3. Decreased Validity of Results
The omission of confounding variables can lead to unexamined bias and decreased validity of research results. Broadcasted to a wider audience, such prejudice may pave the way for dissemination of prejudiced beliefs, unintended consequences, and development of ineffective or harmful policies.
4. Impetus for Innovation
On a more positive note, neglecting confounding variables could serve as an unexpected impetus for innovation, encouraging ingenious statistical methods and experimental designs for detection and management of such factors. Essentially, the overlooked uncontrolled variables could contribute towards the establishment of better methods, improved statistical adjustments, and more thorough experimental designs.
Failing to understand and respect the potential power of confounding variables threatens the very essence of the quest for knowledge. Conversely, recognizing and attending to these variables with methodological dexterity can pave the way to more accurate, ethical, relevant, and ultimately beneficial research. Through correct identification and control of confounding variables, the scientific journey continues towards revealing the complex truth of our world.
Case Studies of Confounding Variables
In the realm of scientific inquiry, rigorous attention to detail is not an option; rather, it is a compulsion. Thus, researchers must uphold precise handling of confounding variables as a pivot around which the entire mechanism of knowledge generation revolves. It is important, then, to look into several case studies that demonstrate the dramatic implications of improperly managed confounding variables.
1. Broken Windows Policing Policy
A notable instance surfaces in the field of criminology. The “broken windows” policing policy, implemented in the 1990s in New York, was based on the belief that reducing minor crimes – notably vandalism – would lead to a decrease in more serious crimes.
Empirical data seemed to support this theory: As minor crime control was tightened, major crime rates went down. However, a confounding variable was overlooked: the simultaneous decline in the city’s unemployment rate. Some critics argue that this overlooked variable may have had more to do with the decrease in crime than the intensified policing.
2. Breastfeeding and Infant Health
In a study aiming to understand the association between breastfeeding and infant health, results initially suggested that breastfeeding led to healthier babies.
However, upon employing a sibling comparison design that controlled for a range of shared genetic and environmental confounders, researchers found that the correlation held only for measures like diarrhea, where confounding is less likely. This turned previously held assumptions on their heads, underscoring that the link between breastfeeding and all aspects of infant health might not be as straightforward as previously concluded.
4. Industrial Pollution and Child Respiratory Illnesses
In a different sphere, environmental science, confounding variables proved to be of paramount importance. A study focused on the link between industrial air pollution and child respiratory illnesses.
After adjusting for multiple confounders, including family history and passive smoking, researchers found that the perceived association between air pollution and respiratory illness was attenuated or reduced. This compelling instance underscores the role of careful control of confounding variables in delineating accurate conclusions.
5. Human Intelligence and Survival Rates
Lastly, in the field of evolutionary psychology, when exploring the link between human intelligence and survival rates, many studies found that those with higher intelligence tended to live longer. However, confounding variables such as the socio-economic status, access to healthcare, and lifestyle choices were not adequately adjusted for.
Subsequent studies controlling for these factors found that the relationship between intelligence and survival was considerably reduced, demonstrating the potential distortion caused by overlooking confounding factors.
These instances underline the issues that confounding variables can precipitate if not diligently controlled. They can twist and turn nascent correlations, leading researchers down paths that deviate significantly from the truth.
Just as confounding variables can be damning, the effort required to identify and control them is equally, if not more, prominent. Recognition of this enduring challenge has crucial implications for researchers and rings alarm bells for the ethical handling of research, ensuring that the path to knowledge remains untainted. The fascinating pursuit of precisely pinpointing how to optimally manage these stealthy infiltrators of research integrity will continue.
Through a thorough exploration of confounding variables, their identification & control techniques, followed by practical examples and compelling case studies, we have established a holistic understanding of this intricate concept. The importance of taking confounding variables into account while designing and analyzing a study can not be overstated. Ignorance of these variables can jeopardize the reliability of the research and cause erroneous conclusions.
Thus, a researcher should always be mindful of potential confounding variables that might influence their study. As we continue to build more complex and sophisticated data models, the role and understanding of confounding variables will only escalate in research.
References
Diallo, A. F., McGlothen‐Bell, K., Lucas, R., Walsh, S., Allen, C., Henderson, W. A., … & McGrath, J. (2020). Feeding modes, duration, and diarrhea in infancy: Continued evidence of the protective effects of breastfeeding. Public Health Nursing, 37(2), 155-160.
Douglas, J. W. B., & Waller, R. E. (1966). Air pollution and respiratory infection in children. British journal of preventive & social medicine, 20(1), 1.
Lamberti, L. M., Fischer Walker, C. L., Noiman, A., Victora, C., & Black, R. E. (2011). Breastfeeding and the risk for diarrhea morbidity and mortality. BMC public health, 11(3), 1-12.
Martorell, R., & O’Gara, C. (1985). Breastfeeding, infant health, and socioeconomic status. Medical Anthropology, 9(2), 173-181.