How can exploratory data analysis help you unravel groundbreaking discoveries using large amounts of data? This article describes the concept, and the outcomes expected of data mining.
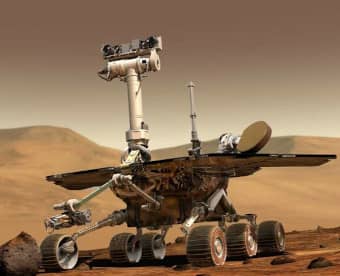
It’s amazing how computers have progressed in the past two decades. State-of-the-art computers can now handle large data sets using developed technologies arising from continuous research and development projects.
As huge amounts of data become available online and even offline, big data analytics comes into play. Now, it’s possible to discover unintended outcomes of data analysis from raw data—the realm of exploratory data analysis.
What are the objectives of exploratory data analysis and what benefits can be gained from this activity? I discuss these two concerns in the next two sections.
Table of Contents
Objectives of Exploratory Data Analysis
The objectives of exploratory data analysis include, but not limited to:
- identifying data outliers,
- identifying trends in time and space,
- detecting patterns of interest,
- generating hypotheses,
- opening opportunities for new ways to collect data, and
- enabling hypothesis testing through experiments.
The Significance of Outliers
In any population of interest, the outliers or those data that deviate from the norm, shows the existence of a segment of that population which may in fact be non-members of that population.
Statisticians usually remove the outliers using certain rules. If they are out of bounds or lying at the extremes of any projected trend in a population, conclusions about the population under study will be affected.
Hence, it will be a good idea to treat the outlier group as part of a different population. If a significant number has been observed. Otherwise, those outliers could be just errors in data input and could be discarded outright.
If indeed there are a lot of outliers, that will open opportunities for closer inspection and interesting discoveries. A Principal Component Analysis would be most appropriate for such data.
Trends in Time and Space
Referred to as spatio-temporal in scientific circles, trends in time and space in the data analyzed allows prediction of outcomes. An example of a spatial trend is a study on the incidence of corruption. The farther the distance from the seat of government, the higher the incidence of corruption (Campante and Do, 2014).
The temporal aspect of data tells us if changes follow a certain direction through time. For example, the price of goods increase by a certain percentage annually, following a curve defined by supply and demand. Exponential population growth significantly influences the price trend through time.
Patterns in Data
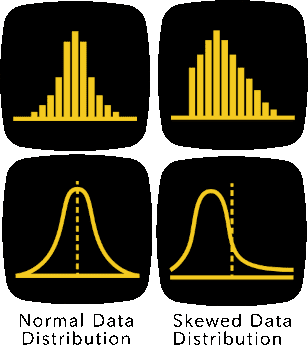
Exploratory data analysis reveals patterns in data in terms of graphical portrayals of data distribution. Statistics describe data patterns as to the center of the distribution, its spread, shape, among other notable features.
For example, the center of distributions follows a symmetric, skewed, or bell-shape (narrow or wide), or other unexpected shapes, such as distributions with gaps or outliers.
Hypotheses Generation
By visual inspection of data, several hypotheses relevant to an organization can be generated. For instance, a decrease in sales trend in a particular part of the year will prompt the data analyst to look into the potential factor or set of factors that influence such a dip.
Major events such as a pandemic would be easy to spot, but two to three factors occurring in the same period require further analysis to discern which one significantly affects the trend. A multiple regression analysis to test the hypothesis whether there is a significant relationship between the multiple factors and sales trend would shed light on the issue.
New Ways to Collect Data
The formulation of hypotheses opens up new data collection opportunities. Additional parameters for data collection expand the current database structure and allow more flexibility in analyzing data. Thus, uncertainty in the outcomes of modeling or prediction is reduced.
Experiments
Experiments can be done to test the findings and incorporating new variables or parameters in the database. For a detailed discussion of these variables and their relevance in doing an exploratory analysis, Prof. Patrick Meyer of the University of Virginia describes and gives examples of the types of variables and how these are used in identifying follow-up statistical data analysis.
Exploratory data analysis reveals new information from a set of data gathered for other purposes. Thus, it maximizes the use of existing data for better organizational management, operational efficiency, modeling for prediction, decision-making, among others based on newly stated objectives.
References
Campante, F. R., & Do, Q. A. (2014). Isolated capital cities, accountability, and corruption: Evidence from US states. American Economic Review, 104(8), 2456-81.
IBM (2021, July 6). What is Exploratory Data Analysis?. Retrieved January 31, 2022 from https://www.ibm.com/cloud/learn/exploratory-data-analysis
© 2022 January 31 P. A. Regoniel
[cite]