What is machine learning? How can this technology help ensure food security in the 21st century and beyond? This article defines machine learning, AI, and algorithms. It explores this technology’s potential to help address food security threats.
Emulation is the best way that people learn. Another way is to teach them to do particular tasks. The same goes for machines. Machines follow what you tell them to do. And mimic your actions like human beings do. This learning method enables the machines—powered by computers—to function like humans using a language that these marvels of technology can understand.
How can machines learn? This question brings us to a growing field of computer technology—artificial intelligence, machine learning, and algorithms.
Let’s see the differences and relationships between these computer terms that baffle newbies in this field.
What is the Difference Between Machine Learning and AI?
Machines do make decisions, almost like humans, based on the data inputted in the computer system that does the processing. This is the essence of machine learning, a domain of artificial intelligence or AI. Computers use a process or set of rules to be followed in calculations by a computer.
Let’s define these computer terms once more and expound on them. I emphasize the key terms that characterize the term. A good understanding of these terms with examples clarifies the differences and will help us understand how machine learning works.
Machine Learning
Machine learning is a subset of artificial intelligence or AI. It refers to the use and development of computer systems that process or follow a set of rules to achieve an objective. That user, customer, executive, or anyone who needs a specific task done defines that objective.
Machine learning refers to the use and development of computer systems that process or follow a set of rules to achieve an objective.
For machines, or to be more accurate—the computer systems, require data input. Computer systems could not process anything without a database.
A database is an organized collection of data that enable computers to work. When large volumes of data are accumulated or gathered, big data analytics will now be possible.
Patterns emerging from data analysis enable data analysts to make inferences or generate hypotheses regarding the relationships of variables selected for analysis.
Thus, we can say that machine learning becomes more efficient and effective when voluminous data has been gathered on a particular issue being studied. The computer can now build statistical models, to predict the outcome given inputs to the system.
Models are useless if you only have a few data available for data analysis. Also, a model fails if it uses erroneous data. Hence, data accuracy, reliability, and integrity are primary considerations in building models. Predictions are much more accurate the bigger the database because it can accommodate all possible data variations for a given parameter or variable.
The following video will further enrich your knowledge about machine learning, artificial intelligence or AI, and algorithms. I explain the last two concepts in the following sections.
Artificial Intelligence or AI
Conceived in 1956 during the Dartmouth Summer Research Project on Artificial Intelligence by McCarthy, artificial intelligence evolved into what it is now. A young British polymath named Alan Turing posed the question: “Why can’t machines use available information as well as a reason just like human beings?”
Thus, the intention was to create machines that can “think” like humans. We can then define Artificial Intelligence as follows:
Artificial intelligence is the human-programmed capability of computers to produce outputs, or perform tasks, that humans normally do through systematic big data processing. It mimics human decision-making via complex computations to meet a desired goal or objective.
Artificial intelligence is the human-programmed capability of computers to produce outputs, or perform tasks, that humans normally do through systematic big data processing.
AI underwent rapid development through the years as computers become cheaper and faster. Now, computers can perform tasks such as assisting in decision-making, translating languages, diagnosing diseases, delivering the right merchandise to the right stores, and recognizing speech. All of these capabilities were achieved using large data sets referred to as big data.
Hence, AI is possible because a lot of data—as necessary inputs to computers—is available for analysis. The realm of big data analytics followed in recent years.
What are Algorithms?
Algorithms make possible the production of outputs by computers. Thus, we can define the algorithm as follows:
An algorithm is the set of rules developed by a knowledgeable computer scientist, engineer, or anyone possessing a good computer background to achieve a particular purpose or outcome. It tells the computer what detailed steps to execute and in what order.
Ananya Rao comprehensively describes and gives examples of algorithm in her post.
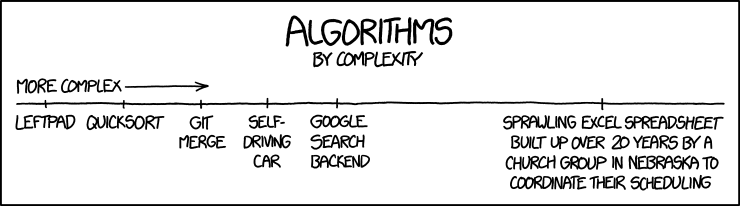
Now, let’s focus on one of the most pressing problems of the world and how machine learning can assist in developing solutions.
Next to the basic needs of humans namely air, and water which we cannot do without, food is a major worldwide concern.
How can technology sustainably feed 7 billion people in the world? This issue is a food security problem.
I discuss this issue critical to human survival in the next section.
Food Security Problem: A Rising Challenge
You always hear the word food security when you get into the issue of exponential population growth. How can agriculture feed an ever-growing population?
Food security is an issue in the past that human technology has overcome over the years, even exceeding expectations and averting the predicted worst-case scenario modeled by the Club of Rome. Modern food production technologies keep up with population demand for food.
I quote the definition of the United Nations’ Committee on World Food Security, thus:
Food security means that all people, at all times, have physical, social, and economic access to sufficient, safe, and nutritious food that meets their food preferences and dietary needs for an active and healthy life.
United Nations’ Committee on World Food Security
But why is the issue of food security always cropping up?
This concern on food security arose because of the threats the following events and economic processes have posed in recent years as well as the future:
- changing climate that reduces food production capacity in many regions of the world;
- conversion of agricultural land into subdivisions, commercial, and industrial areas;
- still growing global population that requires more food;
- limited access to food sources due to poverty;
- pollution that lay waste to agricultural land;
- scarce freshwater supply for farmlands; and
- pest and disease outbreaks.
These driving forces influence food production worldwide at varying degrees of impact. There is a need to incorporate all these factors for successful food production.
While technology has overcome these stressors in the past, will it be able to resolve today’s concerns? Who will win the Mathusian-Cornucopian debate?
Malthusian and Neo-Malthusian adherents view the exponential population growth in a pessimistic perspective with all its negative outcomes like war, famine, poverty, among others. The Cornucopians on the other hand believe that technology will always find solutions to human problems.
Let’s adopt the Cornucopian approach meanwhile and see how cutting-edge technologies can keep up with food security threats as enumerated earlier. We are now in the age where the rise of machines came to the fore. The predictive capacity of computers on quantum leap holds promise in meeting the goals of food security.
Given the worldwide and wide-ranging impacts of environmental and socio-economic as well as political pressures, we cannot always counter and manage all of them despite conscious efforts to do so. Even with technologies at our disposal, we cannot a hundred percent effectively address problematic issues like the ongoing onslaught of the ever-mutating COVID-19 pandemic for more than two years.
But this situation would have been worse without tools that help us cope up and adapt. We are fortunate that our network of computers has aided us significantly in meeting our need for information to better keep up with our food security problems. Well-informed decisions powered by data analytics can resolve the multi-factorial aspects of food production.
Now let’s see how these machines can bring us to a better future.
How Can Machine Learning Help Achieve Food Security?
With the world now reeling due to the effects of COVID-19 on the economy, there is a need to use technology to recover from the pandemic. Of utmost importance is food production potential, as the growing crops had been a perennial problem to farmers due to shifting seasons and the preponderance of pests and diseases due to climate change.
The following video from Google’s Artificial Intelligence development website gives a short background on how artificial intelligence or AI through the application of machine learning can be put to use in improving agriculture.
This story tells us that with more information coming in, it is possible to solve a multitude of problems using machine learning. Greater demand for valuable data to feed computers comes into the picture.
The use of computer technology can enable humankind to adapt and become more resilient to global, regional, and local threats or disturbances. Let’s harness what we got and use artificial intelligence to improve our lives.
© 2021 December 12 P. A. Regoniel
[cite]