In the research and statistics context, what does the term model mean? This article defines what is a model, poses guide questions on how to create one, lists steps on how to construct a model and provides simple examples to clarify points arising from those questions.
One of the interesting things that I particularly like in statistics is the prospect of being able to predict an outcome (referred to as the independent variable) from a set of factors (referred to as the independent variables). A multiple regression equation or a model derived from a set of interrelated variables achieves this end.
The usefulness of a model is determined by how well it can predict the behavior of dependent variables from a set of independent variables.
To clarify the concept, I will describe here an example of a research activity that aimed to develop a multiple regression model from both secondary and primary data sources.
What is a Model?
Before going into a detailed discussion on what is a model, it is always good practice to define what we mean here by a model.
A model, in research and statistics, is a representation of reality using variables that somehow relate with each other. I italicize the word “somehow” here being reminded of the possibility of a correlation between variables when in fact there is no logical connection between them.
A Classic Example of Nonsensical Correlation
A classic example given to illustrate nonsensical correlation is the high correlation between length of hair and height. They found out in a study that if a person has short hair, that person is tall and vice versa.
Actually, the conclusion of that study is spurious because there is no real correlation between length of hair and height. It so happened that men usually have short hair while women have long hair. Men are taller than women. The true variable behind what really determines height is the sex or gender of the individual, not the length of hair.
The model is only an approximation of the likely outcome of things because there will always be errors involved in building it. This is the reason scientists adopt a five percent error (p=0.05) as a standard in making conclusions from statistical computations. There is no such thing as absolute certainty in predicting the probability of a phenomenon.
Things Needed to Construct A Model
In developing a multiple regression model which will be fully described here, you will need to have a clear idea of:
- What is your intention or reason in constructing the model?
- What is the time frame and unit of your analysis?
- What has been done so far in line with the model that you intend to construct?
- What variables would you like to include in your model?
- How would you ensure your model has predictive value?
These questions will guide you towards developing a model that will help you achieve your goal. I explain the expected answers to the above questions. I provide examples to further clarify the points.
1. Purpose in Constructing the Model
Why would you like to have a model in the first place? What would you like to get from it? The objectives of your research, therefore, should be clear enough so that you can derive full benefit from it.
Here, I sought to develop a model. The main purpose is to determine the predictors of the number of published papers produced by the faculty in the university. The major question, therefore, is:
“What are the crucial factors that will motivate the faculty members to engage in research and publish research papers?”
Once the research director of the university, I figured out that the best way to increase the number of research publications is to zero in on those variables that really matter. There are so many variables that will influence the turnout of publications, but which ones do really matter?
A certain number of research publications is required each year, so what should the interventions be to reach those targets? There is a need to identify the reasons for the failure of the faculty members to publish research papers to rectify the problem.
2. Time Frame and Unit of Analysis
You should have a specific time frame on which you should base your analysis from.
There are many considerations in selecting the time frame of the analysis but of foremost importance is the availability of data. For established universities with consistent data collection fields, this poses no problem. But for struggling universities without an established database, it will be much more challenging.
Why do I say consistent data collection fields? If you want to see trends, then the same data must be collected in a series through time.
What do I mean by this?
In the particular case I mentioned, i.e., number of publications, one of the suspected predictors is the time spent by the faculty in administrative work. In a 40-hour work week, how much time do they spend in designated posts such as unit head, department head, or dean? This variable which is a unit of analysis, therefore, should be consistently monitored every semester, for many years for correlation with the number of publications.
How many years should these data be collected?
From what I collect, peer-reviewed publications can be produced normally from two to three years. Hence, the study must cover at least three years of data to log the number of publications produced. That is, if no systematic data collection ensued to supply the study’s data needs.
If data was systematically collected, you can backtrack and get data for as long as you want. It is even possible to compare publication performance before and after implementation of a research policy in the university.
3. Literature Review
You might be guilty of “reinventing the wheel” if you did not take time to review published literature on your specific research concern. Reinventing the wheel means you duplicate the work of others. It is possible that other researchers have already satisfactorily studied the area you are trying to clarify issues on. For this reason, an exhaustive review of literature will enhance the quality and predictive value of your model.
For the model I attempted to make on the number of publications made by the faculty, I bumped on a summary of the predictors made by Bland et al. [1] based on a considerable number of published papers. Below is the model they prepared to sum up the findings.
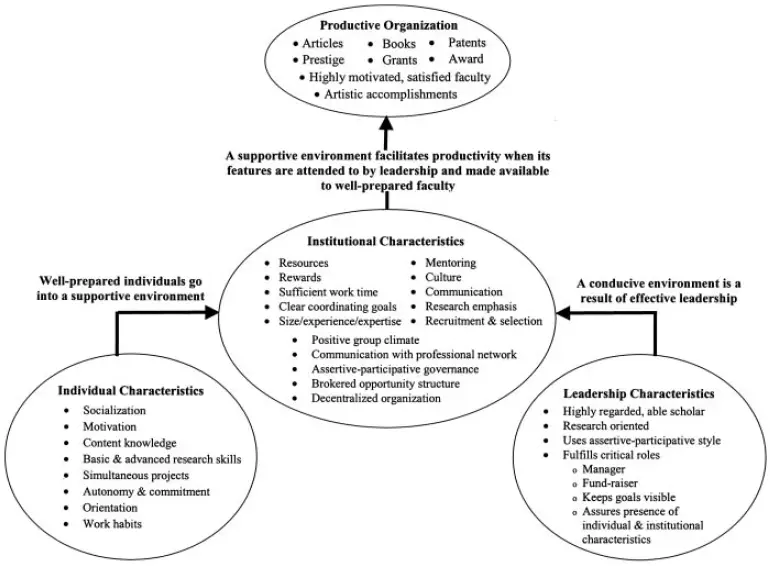
Bland and colleagues found that three major areas determine research productivity namely,
1) the individual’s characteristics,
2) institutional characteristics, and
3) leadership characteristics.
This just means that you cannot just threaten the faculty with the so-called publish and perish policy if the required institutional resources are absent and/or leadership quality is poor.
4. Select the Variables for Study
The model given by Bland and colleagues in the figure above is still too general to allow statistical analysis to take place.
For example, in individual characteristics, how can socialization as a variable be measured? How about motivation?
This requires you to further delve on literature on how to properly measure socialization and motivation, among other variables you are interested in. The dependent variable I reflected productivity in a recent study I conducted with students is the number of total publications, whether these are peer-reviewed.
5. Ensuring the Predictive Value of the Model
The predictive value of a model depends on influence of a set of predictor variables on the dependent variable. How do you determine influence of these variables?
In Bland’s model, we may include all the variables associated with those concepts identified in analyzing data. But of course, this will be costly and time-consuming as there are a lot of variables to consider. Besides, the greater the number of variables you included in your analysis, the more samples you will need to get a good correlation between the predictor variables and the dependent variable.
Stevens [2] recommends a nominal number of 15 cases for one predictor variable. This means that if you want to study 10 variables, you will need at least 150 cases to make your multiple regression model valid in some sense. But of course, the more samples you have, the greater the certainty in predicting outcomes.
Once you have decided on the number of variables you intend to incorporate in your multiple regression model, you will then be able to input your data on a spreadsheet or a statistical software such as SPSS, Statistica, or related software applications. The software application will automatically produce the results for you.
The next concern is how to interpret the results of a model such as the results of a multiple regression analysis. I will consider this topic in my upcoming posts.
Note
A model is only as good as the data used to create it. You must therefore make sure that your data is accurate and reliable for better predictive outcomes.
References
- Bland, C.J., Center, B.A., Finstad, D.A., Risbey, K.R., and J. G. Staples. (2005). A Theoretical, Practical, Predictive Model of Faculty and Department Research Productivity. Academic Medicine, Vol. 80, No. 3, 225-237.
- Stevens, J. 2002. Applied multivariate statistics for the social sciences, 3rd ed. New Jersey: Lawrence Erlbaum Publishers. p. 72.
Updated May 6, 2022 © P. A. Regoniel