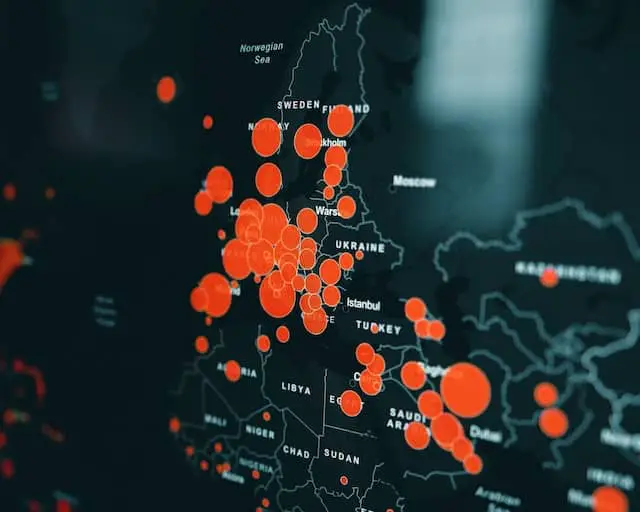
What is statistical sampling? Why is it important in conducting research? This article explains the concept and significance of statistical sampling, the key techniques, and 6 examples of its practical application.
Table of Contents
Introduction
Statistical sampling, a critical concept in the sphere of research, serves as the backbone for potential decisions derived from data analysis. This method is integral to gaining knowledge about population characteristics, validating hypotheses, and helps drive accurate decision-making processes.
The significance of statistical sampling, its key techniques, practical applications, and the challenges and limitations associated with it encapsulate the core of this comprehensive discussion.
Notably, the various methods incorporated in statistical sampling – including random sampling, stratified sampling, cluster sampling, and systematic sampling, all have unique elements of methodology, application, advantages and disadvantages, tailored in accordance with the nature of data and study in question.
Before doing the calculations, however, it is important that you know fully well the purpose of statistical sampling and why it is an important part of the research process.
This article aims to provide you a good understanding of these concepts, coupled with their real-world applications in fields such as economics, sociology, psychology, public health, and market research.
Concept and Significance of Statistical Sampling
Why is statistical sampling a crucial tool in scientific research?
The concept of statistical sampling refers to the practice of selecting a subset of individuals from a larger population, with the goal of using characteristics, behaviors, or traits of the sample to make inferences about the larger group from which it is derived. This method is indispensable within scientific investigation, allowing for accurate estimates about phenomena without necessitating a full survey or inquiry of an entire population.
Even casual observance of the world reveals the impracticality, and often impossibility, of evaluating every single individual or object within a given set. For instance, consider trying to determine the average height of all human beings on Earth (8.1 billion at this moment and population growth is dynamic) or the average temperature of the Pacific Ocean.
The impossibility of collecting data from each member under such circumstances necessitates a measure that can efficiently facilitate these evaluations on a smaller, yet representative scale. This method is none other than statistical sampling.
By taking a sample, researchers can make broad predictions, or estimates, about parameters of interest in large datasets. To ensure that the sample is representative of the intended population, several techniques may be employed, such as simple random sampling, systematic sampling, stratified sampling, and cluster sampling. These techniques help in minimizing sampling errors – the difference between the results obtained from the sample and the actual result that would have been achieved had the entire population been considered.
The concept of statistical sampling also serves as a bedrock of confidence intervals and hypothesis testing – two integral aspects of inferential statistics. Confidence intervals utilize sampling distribution to provide a range within which the true population parameter falls with a specific level of confidence. Hypothesis testing is another application of statistical sampling that helps in making decisions about the population by studying the sample.
Statistical sampling is crucial in scientific research due to its efficiency and cost-effectiveness. This strategy significantly reduces the amount of work, time, and expenses involved in data collection. Moreover, it makes large-scale data-based studies feasible, which precisely and accurately could help in understanding phenomena and making informed decisions. Besides this, the statistical inference drawn from sampling better preserves the integrity of the population under study, like certain biomedical research where repetitive testing on the population could be harmful.
It is clear that statistical sampling serves as a fundamental strategy in the domain of scientific investigation. Without it, important insights would remain hidden in a sea of unmanageable data, and the breadth and depth of our understanding with the world around would be all the poorer.
Thus, the concept of statistical sampling is not just a strategy or method; it is, in essence, a lifeline for scientific research, valued in its pursuit to uncover the mysteries of the universe.
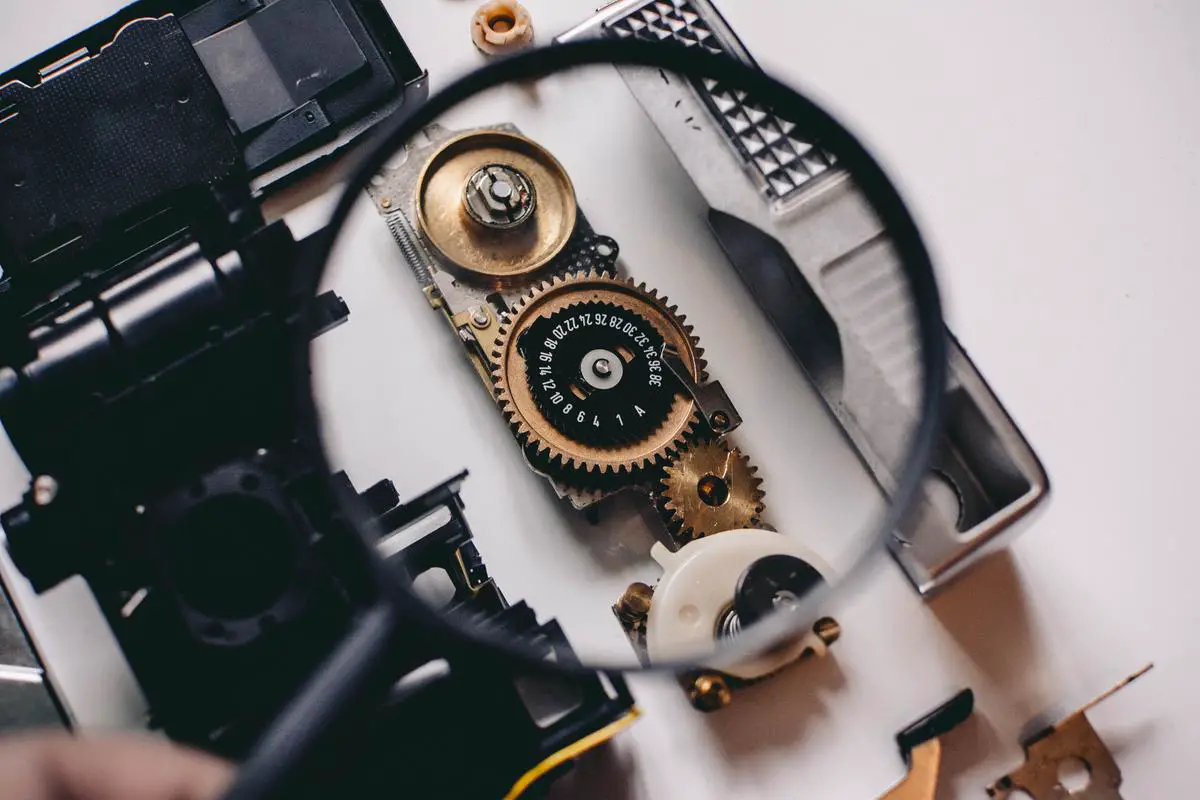
Key Techniques Involved in Statistical Sampling
Prominently, in the realm of statistical sampling, the cornerstone techniques enlisted comprise Simple Random Sampling, Stratified Sampling, Cluster Sampling, and Systematic Sampling. Each approach provides an essential methodology within the statistical landscape, with their specialization securing particular utilities across various research scenarios.
1. Simple Random Sampling
Simple Random Sampling is the most fundamental, yet critical technique that offers unparalleled objectivity in its execution. Each member of a population possesses an equal opportunity for inclusion in the sample space, thereby fostering the potential for priceless neutrality in statistical inferences. Its usage reigns supreme within research fields that emphasize equal representation and randomization within their experimental design – quite often seen within clinical trials, opinion polls, and wide-scale surveys.
2. Stratified Sampling
Transitioning to Stratified Sampling, it is characterized by the division of the target population into non-overlapping subgroups or ‘strata’ based on shared characteristics. This approach ensures that each stratum is accurately represented in the sample, thereby reducing sampling error.
The applicative power of Stratified Sampling is most detectable within domains that underline the role of socio-demographic factors, questioning the varying impacts within distinguishable subgroups. For instance, studying disease prevalence across gender, age, or income categories often employs this method for its insightful capture of population diversity. The strata of gender, for example, will be male and female. Randomly sampling from males, then females, will strike a balance in the number of male and female participants of a study. Hence, researcher bias is avoided.
3. Cluster Sampling
Cluster Sampling, strikingly, deconstructs the population into separate ‘clusters’, usually along geographical lines. Thereafter, clusters are randomly selected for data collection.
The advantage of cluster sampling emerges in studies of geographically large or logistically complex situations, enabling researchers to bypass the exhaustive barrier of covering all individuals within a population. It is applicable in nation-wide public health surveys or environmental studies, where it tempers the logistical complexities whilst ensuring sound statistical inference.
4. Systematic Sampling
Systematic Sampling presents an ordered approach where every nth member of a population is selected, subsequent to a randomized starting point. The technique splendidly balances the objectivity of simple random sampling and the convenience of choosing subjects in a systematic fashion. When processing quality control, manufacturing audits, or periodic surveys, systematic sampling performs optimally due to its efficient amalgamation of randomness and order.
Strategically applying each principle when designing research best explores the statistical landscape in its entirety, ensuring the most appropriate sampling method is utilized. Thus, it is necessary to ponder on the intended samples of the study to choose the correct statistical sampling technique to use.
Practical Applications of Statistical Sampling: 6 Examples
1. Market Research
One particular application of statistical sampling is market research. Businesses harness the power of statistical sampling in the investigation of consumer behavior.
By dissecting broad market into a representatively smaller subset, researchers are able to identify trends and patterns, aiding in the prediction of prevailing consumer choices. This information contributes significantly to the direction of product design, the hours of service, sales strategies, and promotional tactics. Where it would be unworkable to query every potential consumer, statistical sampling paves a pragmatic pathway to comprehending the intricacies of the market.
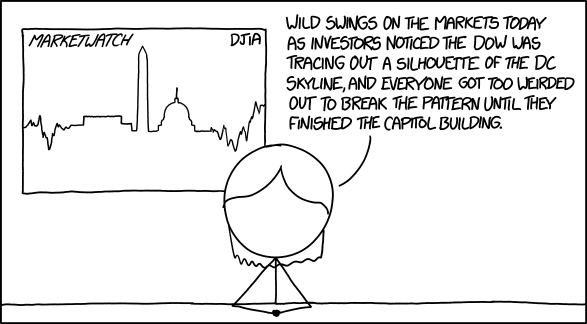
2. Public Health
In the public health sector, statistical sampling stands as an invaluable tool. Public health organizations use statistical sampling to monitor the spread of diseases, keep a check on health trends, and measure the success of health interventions.
Population health surveys often rely on statistical sampling to provide an astute snapshot of health prevalence rates. Data collected through such methods then serve as inputs for disease surveillance systems, national health policies, and strategic health interventions.
3. Census
The census, that mammoth undertaking of population data enumeration, frequents the use of statistical sampling. Across the globe, nations find it pragmatically impossible to attain complete counts of their populations accurately.
Hence, reliable statistical sampling methods are employed to estimate various demographic characteristics – age, sex, employment, migration patterns, and more. These estimates are then scaled to match the total population, guiding policy-making, resource allocation, and socio-economic planning at a national level.
4. Quality Control and Manufacturing
In the realm of quality control and manufacturing, statistical sampling serves as a vital cog as well. Complete analyses of all production units would be cost-prohibitive and time-consuming in large-scale manufacturing.
To overcome the cost concern, batches of products are tested as a representative sample, allowing gaps in quality to be identified and rectified. This ensures the reliability of the product while keeping the testing methodology feasible and economical.
5. Meteorology
Staying true to its versatile nature, statistical sampling is also valuable the realm of meteorology. In weather forecasting, it forms the groundwork for climate models and weather simulations.
Random samples of meteorological data points—temperature, humidity, barometric pressure, wind speed—captured from various geographical and temporal scales, contribute to the development of accurate weather predictions.
6. Education
The educational arena is another significant beneficiary of statistical sampling. Educational assessments, both at the national and international spectrum, rely on it to gauge the effectiveness of learning strategies, curriculum developments, and policy interventions. Mining insights about student performances from a group of candidates, rather than an entire cohort, allows for the identification of areas of improvement, guiding future pedagogical strategies.
While the science behind statistical sampling is intricate, it is the practical application of these methods that truly honors the intricate dance between numbers, patterns, and life.
Despite the power of statistical sampling, we should also recognize its challenges and limitations. I discuss this further in the next section.
Challenges and Limitations of Statistical Sampling
Despite the apparent flexibility and usability of statistical sampling, it is essential to underscore the many challenges and limitations that the application encounters in various facets.
1. Unreliability of Non-Probability Sampling Methods
Of paramount significance is the concept of probability. Given that statistical sampling hinges on probability, non-probability sampling methods pose a challenge.
One such method is convenience sampling, where selections are determined by their accessibility or convenience. This approach introduces selection bias, thereby undermining the representative nature of the sample used and inflicting errors on outcomes.
2. Population Complexity
The inherent complexity of populations can present another challenge.
Frequently, populations are heterogeneous, full of diverse individuals harboring distinct characteristics. In the application of statistical sampling, it can be laborious to categorize these numerous categories aptly.
This challenge underlines the importance of a method like stratified sampling—previously mentioned—but also the additional labor and costs involved in accurately defining and accessing these diverse strata.
3. Sampling Bias
Sampling bias, another daunting limitation, can be subtle yet devastatingly impactful on the research’s credibility. Sampling bias occurs when randomization is not achieved or is incomplete, causing the sample to improperly represent the target population. How the sample is selected can directly influence whether the subsequent data can be effectively used in generalizing for the larger population.
4. Hawthorne Effect
Besides, the ‘observer effect’ or ‘Hawthorne effect‘ emerges across the spectrum. This term refers to the alteration of individuals’ behavior due to the consciousness of being observed or studied. It presents a more latent challenge in applying statistical sampling, particularly in fields such as social sciences and public health monitoring.
5. Non-Response Bias
Non-response bias is another notable limitation. A part of the target group might refuse to participate or be unreachable, thereby creating a sample that does not fully represent the population.
This problem is often encountered in areas as diverse as market research, educational assessments, and census data estimation. Where response rates dramatically differ among various groups within a population, the issue exacerbates.
6. Size of Sample Population
The size of the sample population also harbors challenges. Larger samples might offer considerable data, but they also necessitate greater resources in terms of time, manpower, and finances. Conversely, smaller samples might be less resource-intensive but may fail to represent the population adequately, leading to erroneous conclusions.
While the merits and utility of statistical sampling are undeniable, grappling with its challenges and limitations, such as sampling bias, non-response bias, and the complex heterogeneity of populations, is crucial. By acknowledging these constraints, statisticians—be they in meteorology or manufacturing—are better equipped to handle issues and improve their ability to make sound inferences about their respective populations.
Read more: How is sample size calculated?
Conclusion
As we discuss the merits of statistical sampling, recognizing its challenges and limitations is equally vital. Researchers often grapple with hurdles like bias and variability, which can potentially impact the reliability of data and its subsequent interpretation.
Although these drawbacks pose significant risks, effective strategies can be implemented to mitigate these limitations, enhancing the robustness of research outcomes.