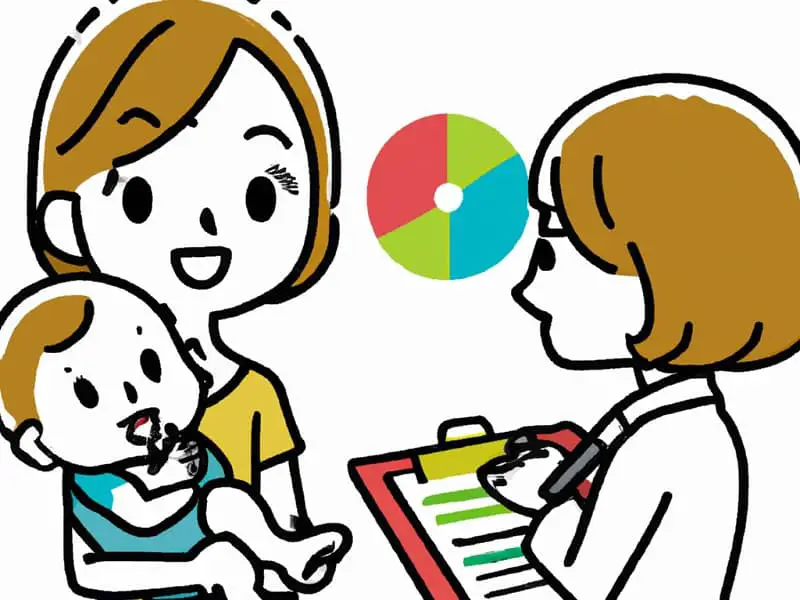
Analyzing data in qualitative research requires six steps. Read on to find out.
Table of Contents
Introduction
Data analysis is a crucial step in any research process, as it helps to make sense of the collected data and draw meaningful conclusions. However, in qualitative research, the methods and approaches used for data analysis differ from those in quantitative research.
This article aims to provide guidance specifically for researchers engaged in qualitative research.
Unlike quantitative research, where data analysis often involves statistical techniques and numerical data, qualitative research focuses on understanding the meaning and interpretation of data. Qualitative data analysis involves a more subjective and interpretive approach, as it seeks to uncover patterns, themes, and insights within the data.
Qualitative researchers often use techniques such as coding, thematic analysis, and constant comparison to analyze their data. These methods allow researchers to identify recurring patterns, extract key themes, and explore the relationships between different elements of the data.
By analyzing qualitative data, researchers can gain a deeper understanding of the phenomena they are studying and generate rich and nuanced insights. It is important to note that data itself is without meaning until it is analyzed. Therefore, the process of data analysis is essential in qualitative research to derive meaningful interpretations and contribute to the existing body of knowledge.
In the following sections, we will explore the steps involved in analyzing data in qualitative research and discuss some common pitfalls to avoid. By following these guidelines, researchers can enhance the rigor and validity of their qualitative research findings.
How to Analyze Data in Qualitative Research
Analyzing data in qualitative research involves a systematic and rigorous process to make sense of the collected data and derive meaningful interpretations. By saying rigorous here means the process of doing the analysis is careful and detailed.
In this section, we will explore the steps involved in analyzing data in qualitative research and explain why each step is required. By following these steps, researchers can ensure the rigor and validity of their findings.
Step 1: Data Preparation
The first step in analyzing qualitative data is to prepare the data for analysis. This involves organizing and structuring the data in a way that facilitates analysis.
What should the researchers do?
Researchers should transcribe interviews, group similar data together, and ensure that the data is easily accessible for further analysis. Transcription is a tedious process as it would take hours to listen to interviews and write them to make sense.
Data preparation is crucial as it sets the foundation for the subsequent steps and ensures that the data is ready for analysis.
Step 2: Familiarization
Once the data is prepared, researchers need to become familiar with the data. This step involves reading and re-reading the data to gain a comprehensive understanding of the content. Thus, qualitative researchers require way lesser number of data sources because it takes considerable time to figure out each data set.
Qualitative researchers should immerse themselves in the data, taking notes, and identifying initial impressions and patterns. Familiarization helps researchers to develop a deep understanding of the data and identify potential themes or patterns that may emerge. It takes skill and a good background or understanding of the topic at hand.
Step 3: Coding
Coding is a fundamental step in qualitative data analysis. It involves systematically categorizing and labeling segments of data based on their content or meaning.
Researchers assign codes to different parts of the data to identify themes, concepts, or ideas. Coding helps to organize the data and make it more manageable for further analysis. It also allows researchers to identify similarities, differences, and relationships within the data.
Step 4: Theme Development
Once the data is coded, researchers can start developing themes. Themes are overarching patterns or concepts that emerge from the data.
Researchers identify commonalities, recurring ideas, or significant findings within the coded data and group them into themes. Themes provide a way to organize and interpret the data, allowing researchers to make connections and draw meaningful conclusions.
A mind map would help the researcher understand the relationships between the different themes.
Step 5: Data Exploration
After developing themes, researchers engage in a process of data exploration. This involves looking further into the data to uncover additional insights.
Researchers may revisit the data, conduct further analysis, or seek additional perspectives to gain a comprehensive understanding of the phenomena under study. It pays, then, to have a colleague see the findings and get their viewpoints on the matter.
Data exploration helps to ensure that researchers have considered all relevant aspects of the data and have captured the richness and complexity of the research topic.
Step 6: Interpretation
The final step in analyzing qualitative data is interpretation. This involves making sense of the data, drawing conclusions, and generating insights.
Researchers critically analyze the themes and patterns identified in the data, considering their implications and significance. Interpretation requires researchers to reflect on the data in relation to the research questions or objectives and to consider alternative explanations or perspectives. It is through interpretation that researchers derive meaningful insights and contribute to the existing body of knowledge.
Each of these steps is essential in analyzing data in qualitative research. Data preparation ensures that the data is ready for analysis, while familiarization helps researchers develop a deep understanding of the data. Coding and theme development provide a structure for organizing and interpreting the data, while data exploration ensures a comprehensive analysis. Finally, interpretation allows researchers to draw meaningful conclusions and generate insights.
By following these steps, researchers can navigate the complex process of analyzing qualitative data and derive rich and nuanced findings.
It is important to note that these steps are not necessarily linear and may involve iteration (repeating the process) and revisiting previous steps. The iterative nature of qualitative data analysis allows researchers to refine their analysis and ensure the rigor and validity of their findings.
Some Pitfalls in Analyzing Data in Qualitative Research
While analyzing data in qualitative research can provide valuable insights, there are certain pitfalls that researchers should be aware of. Understanding these pitfalls and taking appropriate actions can help minimize or even eliminate the weaknesses associated with qualitative research.
In this section, we will discuss some important things to consider when analyzing data in qualitative research. Recommendations are offered to address these challenges.
1. Subjectivity
One of the main pitfalls in qualitative research is the potential for subjectivity. Since qualitative data analysis involves interpretation and judgment, researchers’ biases and preconceived notions can influence the analysis process.
To address this, researchers should strive for transparency and reflexivity. They should document their assumptions, beliefs, and perspectives throughout the analysis process, allowing for a more objective and transparent interpretation of the data.
2. Lack of Generalizability
Another challenge in qualitative research is the limited generalizability of findings. Qualitative research often focuses on specific contexts or individuals, making it difficult to apply the findings to a broader population.
To mitigate this limitation, researchers can employ purposive sampling techniques to ensure diversity in their sample. Additionally, researchers should provide rich descriptions of the research context and participants, allowing readers to assess the transferability of the findings to their own contexts.
3. Time and Resource Intensive
Analyzing qualitative data can be a time and resource-intensive process. The iterative nature of qualitative analysis, involving multiple rounds of coding, theme development, and interpretation, requires significant time and effort.
To manage this challenge, researchers should plan their analysis process carefully, allocating sufficient time and resources. They can also consider using qualitative data analysis software to streamline and facilitate the analysis process.
4. Data Overload
Qualitative research often generates a large volume of data, which can be overwhelming to analyze. Researchers may struggle to identify relevant themes or patterns amidst the vast amount of data.
To address this, researchers should adopt a systematic approach to data analysis, breaking down the data into manageable segments. They can also use data visualization techniques, such as charts or diagrams, to aid in the identification and organization of themes.
5. Lack of Consistency
Maintaining consistency in qualitative data analysis can be challenging, especially when multiple researchers are involved. Differences in coding or interpretation can lead to inconsistencies in the analysis process and findings.
To ensure consistency, researchers should establish clear coding guidelines and conduct regular meetings or discussions to address any discrepancies. Inter-rater reliability tests can also be conducted to assess the consistency of coding across researchers.
By considering these pitfalls and implementing the recommended actions, researchers can enhance the rigor and validity of their qualitative data analysis. Transparency, reflexivity, purposive sampling, careful planning, systematic analysis, and consistency are key factors in minimizing the weaknesses associated with qualitative research. While qualitative research has its limitations, addressing these pitfalls can contribute to the generation of meaningful and reliable findings.
Conclusion
In conclusion, when analyzing qualitative data, researchers should be mindful of subjectivity, limited generalizability, time and resource constraints, data overload, and the importance of consistency. By considering these factors and implementing the recommended actions, researchers can enhance the rigor and validity of their qualitative data analysis. These considerations contribute to the generation of meaningful and reliable findings in qualitative research.
Useful References
Dey, I. (2003). Qualitative data analysis: A user friendly guide for social scientists. Routledge.
Miles, M. B., & Huberman, A. M. (1994). Qualitative data analysis: An expanded sourcebook. Sage.
Timmermans, S., & Tavory, I. (2022). Data analysis in qualitative research: Theorizing with abductive analysis. University of Chicago Press.