Statistical variance is an often-encountered word in statistics that warrant explanation for better student appreciation and understanding. This article elucidates the principles and assumptions behind this concept. Read on to see how a better understanding of statistical variance will help you understand the data that you have gathered in a more meaningful way.
Understanding the intricacies of statistical variance unveils the secrets behind data interpretation. Statistical variance, a cornerstone in the study of statistics, plays a major role in determining how data spreads around the mean value.
This introductory exploration delves into the foundation of variance, the principles that underpin it, and the mathematical maneuvers involved in its disaggregation. The journey takes us through the varied significance and widespread applications of variance, traversing an array of disciplines from finance to physics.
As we delve further, the potential pitfalls and limitations of variance are not left untouched, offering an unbiased and holistic view of this fundamental statistical tool.
Table of Contents
Definition of Statistical Variance
Statistical Variance in the Realms of Statistical Science
Statistical variance is an integral and fascinating concept in the realm of statistical analysis frequently employed to quantify the extent of spread within a dataset. A highly engaging measure from the heart of statistics, variance gauges the dispersion of a set of data points from their mean. Built on the foundation of squared deviations, the essence of variance plunges into a deeper understanding of data variability than a straightforward average.
The variance is computed by calculating the mean of squared differences of each data point from the overall mean. In simpler terms, variance suggests how data is scattered in a distribution. It provides a clearer perception of uncertainty, allowing a comprehensive view of variability, essential for meticulous statistical analysis.
Interestingly, when we square the standard deviation (another measure of dispersion), we obtain variance. This relationship also serves to highlight variance’s importance, given its contribution to understanding data distribution.
Quite importantly, it must be acknowledged that variance isn’t necessarily an intuitive concept, for it deals with squared units which might pose interpretation challenges. It does, however, excel at functioning as a “launch pad” for more interpretable measures such as its square root counterpart, the standard deviation.
When elucidating the relevance of variance, one notes its pivotal role in inferential statistics, including Hypothesis Testing and Confidence Intervals. Assumptions regarding variance significantly influence results of these critical procedures, shaping foundational aspects of decision-making in various domains, including healthcare, social sciences, economics, and more. For instance, in finance, portfolio variance is used to examine the volatility of multiple assets, thereby guiding investment strategies.
Moreover, in areas such as Machine Learning and Statistical Modeling, variance offers insight into the prediction error and model robustness. It plays a seminal role in defining “overfitting” and “underfitting”, phenomena that can seriously impact model performance. Therefore, mastering variance allows statistical professionals to optimize their methodologies, thereby enhancing the efficiency and reliability of their studies or predictions.
Within the realm of normal distribution, variance comes to the forefront, vital for the interpretation and understanding of a lot of biological and social phenomena, further extending its usefulness. Building up from this, the famous “68-95-99.7” rule or empirical rule rattles off percentages of data lying within one, two, or three standard deviations from the mean, underlining again the relationship between variance and the standard deviation.
From an overarching viewpoint, the essence of statistical variance is an indispensable lens to gauge the degree of data spread, shaping the core of intensive statistical analysis. It defines data variability, whose knowledge indeed empowers scientific pursuits, enabling a holistic understanding of data and guiding statistical inference across myriads of sectors and applications.
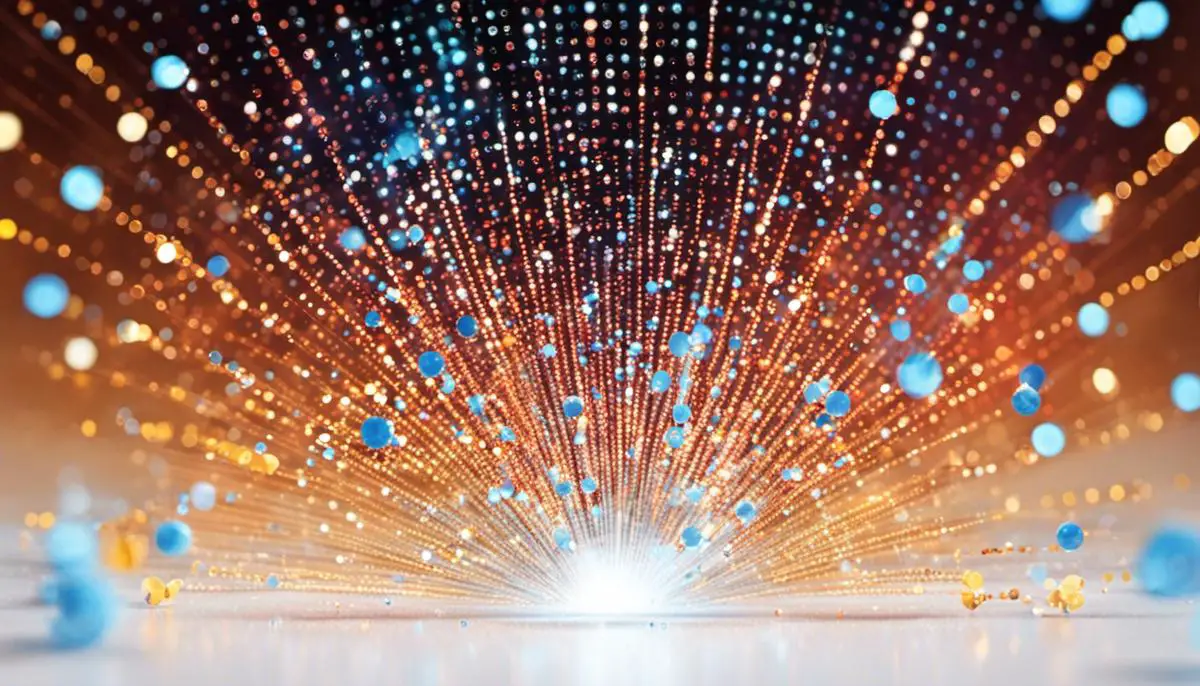
6 Principles and Assumptions behind Variance
The elucidation of statistical variance rests primarily upon its key principles and core presumptions that form the cornerstone of quantitative research. From data stream studies to Big Data analyses, the intrinsic value of variance operates under several guiding principles; including probabilistic reasoning, the law of large numbers, and the significance of sample size.
1. Probabilistic Reasoning
Probabilistic reasoning underpins the function of variance, providing a framework for interpreting the predictability of random events. This principle enables the prediction of outcomes, not as definite certainties, but as probabilities within a range of possibilities. Its extrapolation as statistical variance permits the quantification of data spread, demystifying the unpredictability associated with raw unidimensional data.
2. Law of Large Numbers
Equally inherent to understanding variance is the Law of Large Numbers which postulates that as a sample size grows, the sample mean tends to approach the population mean. This law elucidates an inverse relationship between variance and sample size, that is, larger sample sizes typically yield lower variances, thus bringing forth more reliable estimates and a finer picture of the population.
3. Principle of Sampling Distribution of Variance
Principle of Sampling Distribution of Variance, another guiding principle, decrees that provided the population from which the sample is taken is normally distributed, the distribution of the sample variance approximates the chi-squared distribution – a vital underpinning for the usability of variance in statistical tests.
4. Assumption of Homoscedacity
The presuppositions of variance, on the other hand, underscore the need for datasets to exhibit certain characteristics for variance to serve as a valid measure. The assumption of homoscedasticity is pivotal here, demanding the variability in scores for one variable is roughly the same at all values of another variable. In essence, in a scatterplot of variables, the dots must be equally scattered across all value ranges.
5. Necessity for Numeric Data
Another presupposition, the Necessity for Numeric Data, posits that statistical variance is only applicable for numeric or continuous data, assisting to decode diversity within such data sets.
6. Independence of Observations
Variance also predicates on the condition of Independence of Observations. The measurements on different experimental units are assumed to be independent of each other – an outlier in one observation should not directly influence an outlier in another.
In summation, these guiding principles and presuppositions provide a robust theoretical backbone to statistical variance and its relevance in quantitative analyses. They enable variance to paint a more comprehensive picture of data dispersion, supporting the employability of this measure across a bouquet of scientific and commercial ventures. It is upon these tenets that the expansive utility and applicability of statistical variance is predominantly based, contributing to turning raw, unprocessed data into functional, actionable intelligence.
Calculation of Variance
While the preliminary understanding of variance delves into its calculation and interpretation, it is as equally crucial to acknowledge that variance serves as an empirical bridge between theorems and real-world applications. In order to establish a solid foundation for subsequent analyses, exploring the roots of variance through the lens of probabilistic reasoning is vital.
Just as any other statistical concept, variance stems from probabilistic reasoning implemented to interpret random occurrences. The essence of this reasoning is the belief that due to randomness permeating the world, precise prediction is out of reach. Nevertheless, probabilistic reasoning enables capturing succinct and relevant aspects of any random phenomenon.
Variance witnesses its substantial use in forming this reasoned judgement about uncertain events by quantifying the probable departure from the true unknown. It essentially measures the degree to which a set of data points are spread out from the average value, thereby introducing precision into inferences about uncertain phenomena.
Variance essentially measures the degree to which a set of data points are spread out from the average value, thereby introducing precision into inferences about uncertain phenomena.
Pitfalls and Limitations of Variance
Despite the far-reaching benefits and numerous applications of statistical variance, it is important to remain conscious of its potential pitfalls and limitations.
Nature of Outliers
One primary concern with the usage of variance pertains to the nature of outliers. In the world of statistics, an outlier is an observation that significantly deviates from other observations in the dataset.
While every datum contributes to the variance, outliers have a profound sway over its value. This is because variance considers the square of deviations, a formula that amplifies outlier influence. Consequently, a single, substantial outlier can inadvertently inflate variance, potentially leading to misinterpretation or misrepresentation of the full dataset’s variability.
Variance Exclusively Applies to Interval or Ratio Datasets
Next, variance as a measure of dispersion exclusively applies to datasets of the interval or ratio variety; categorical or ordinal datasets are rendered insignificant. This is because calculation of variance entails the manipulation of actual numbers. Any vain attempt to calculate the variance of non-numerical datasets might distort results or lead to erroneous conclusions.
Tendency to Obscure Directionality
Another crucial consideration tied to variance is its tendency to obscure the directionality or sense of distribution. Variance, describing the divergence of individual data points from their mean, inherently undermines the presence of any skewness in the dataset. This caveat necessitates the use of additional statistical tools such as skewness measures for comprehensive statistical analyses, undermining the standalone utility of variance.
Variance Assumes Identical Distribution
Furthermore, variance analysis assumes identical distribution or homogeneity across groups or samples. This homoscedasticity prerequisite limits its utility in heterogeneous datasets, restrictive in particular for cross-cultural social research or ecological studies where variation across groups is an intrinsic component.
Subject to Misinterpretation
Finally, variance often poses conceptual challenges due to its squared units of measure, potentially leading to confusion or misinterpretation. While standard deviation partially rectifies this by providing a measure in the original units, the issue persists when variance is employed in subsequent calculations or modelling.
Key Takeaways
Variance, despite its integral role in statistics and research methodology, is not a panacea. A thoughtful approach to statistical analysis often requires the selection and utilization of several tools and measures, with variance serving as one piece in the larger puzzle of incisive, data-driven comprehension. It is through the holistic integration of such statistical tools, with the awareness of their limitations, that we can fruitfully navigate the dynamic realm of data analysis.
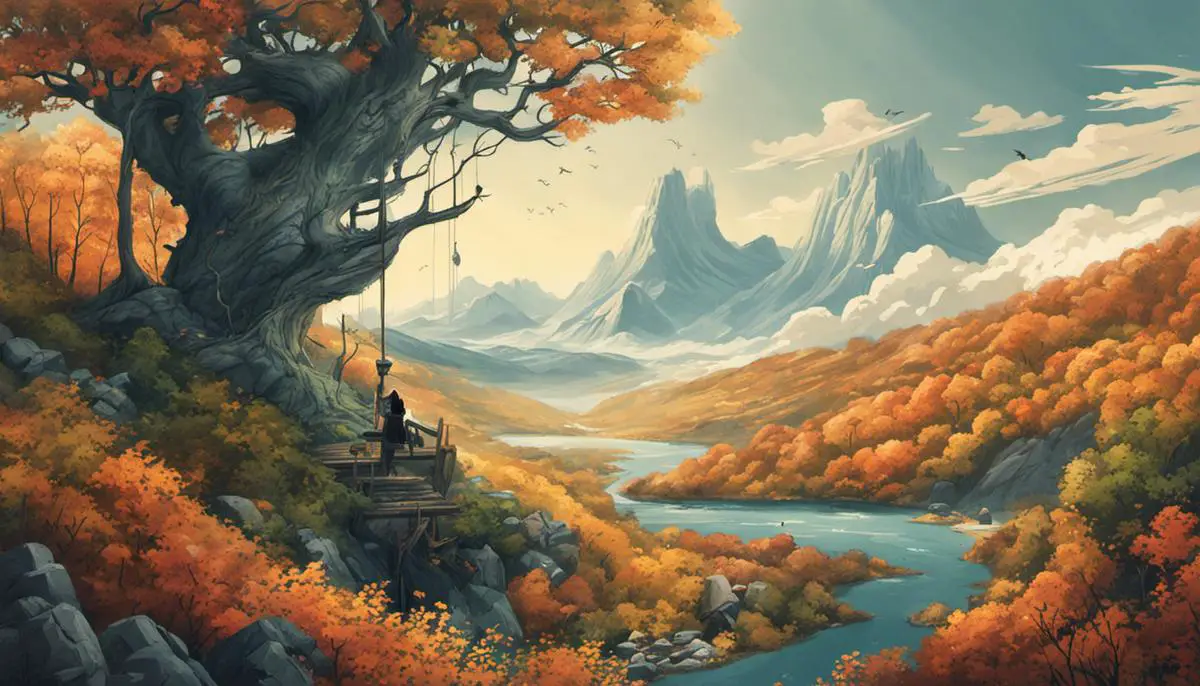
With a thorough understanding of statistical variance, one gains the ability to optimally manage risks, make informed decisions, and construct robust statistical models. Yet, it’s essential to approach the concept of variance with balanced viewpoints, acknowledging its limitations and vulnerabilities to extreme values, coupled with the challenges it poses during interpretation.
Embracing statistical variance with all its intricacies not only enhances one’s quantitative research skills but also fosters a deeper appreciation for the beautiful complexities that underline our data-driven world.